使用python scrapy爬取全国小区信息,并保存至mysql和Excel
小白第一个项目,还望各位大佬斧正!!!### 目标
此次爬取的网站是楼盘网,因为只爬取小区信息,所以先从深圳小区(http://sz.loupan.com/community/)网页入手分析,然后发散爬取至全国。
爬取的信息包括省,所属市,所属区,小区名,小区链接,详细地址,经纬度,交通,价格,物业类型,物业价格,面积,户数,竣工时间,车位数,容积率,绿化率,物业公司,开发商。
保存至Excel和mysql,也可以保存至MongoDB,看个人需求。
#### 字段目录
!(https://img-blog.csdnimg.cn/20200327155924261.png?x-oss-process=image/watermark,type_ZmFuZ3poZW5naGVpdGk,shadow_10,text_aHR0cHM6Ly9ibG9nLmNzZG4ubmV0L3UwMTI0MjQzMTM=,size_16,color_FFFFFF,t_70)
### 效果
#### mysql表:
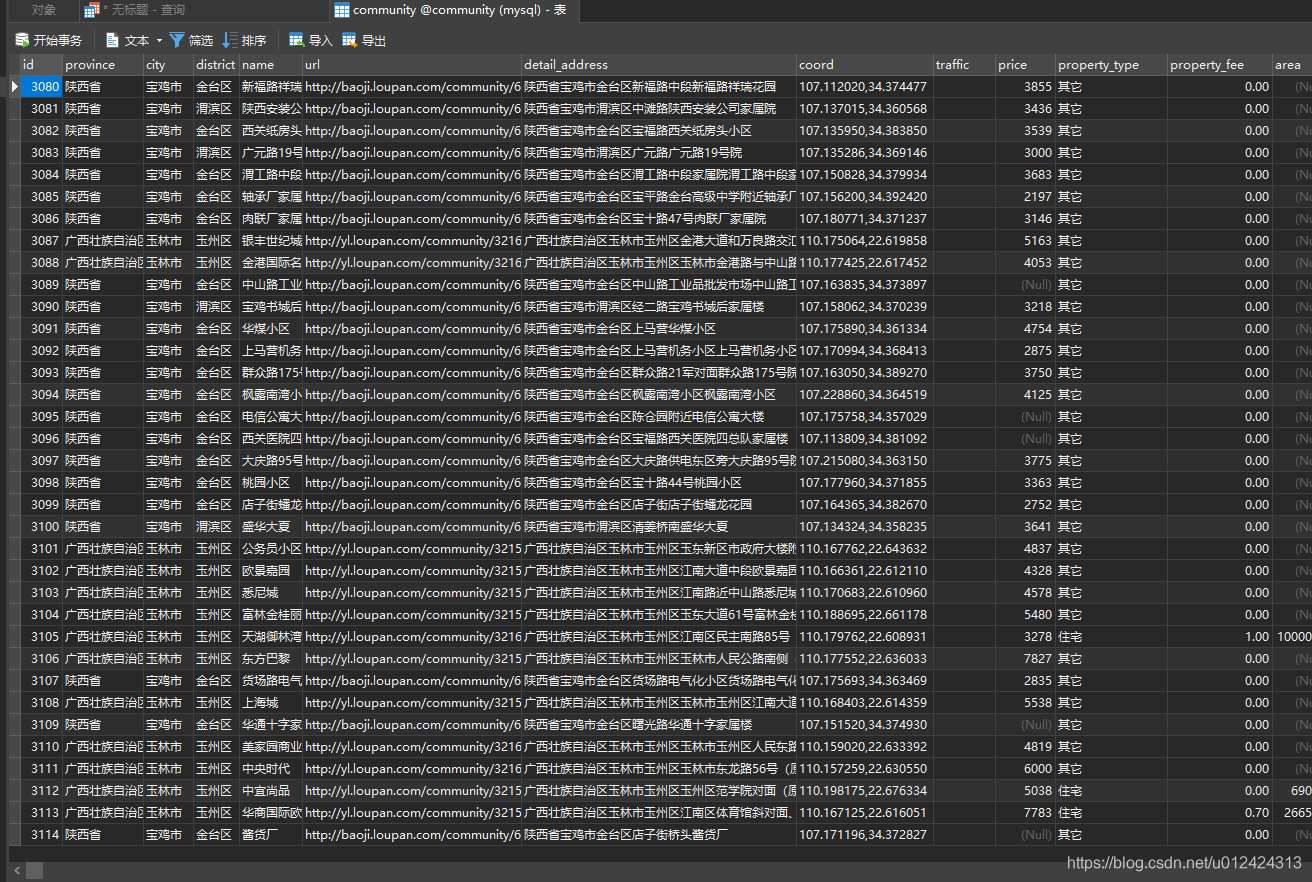
#### excel表:
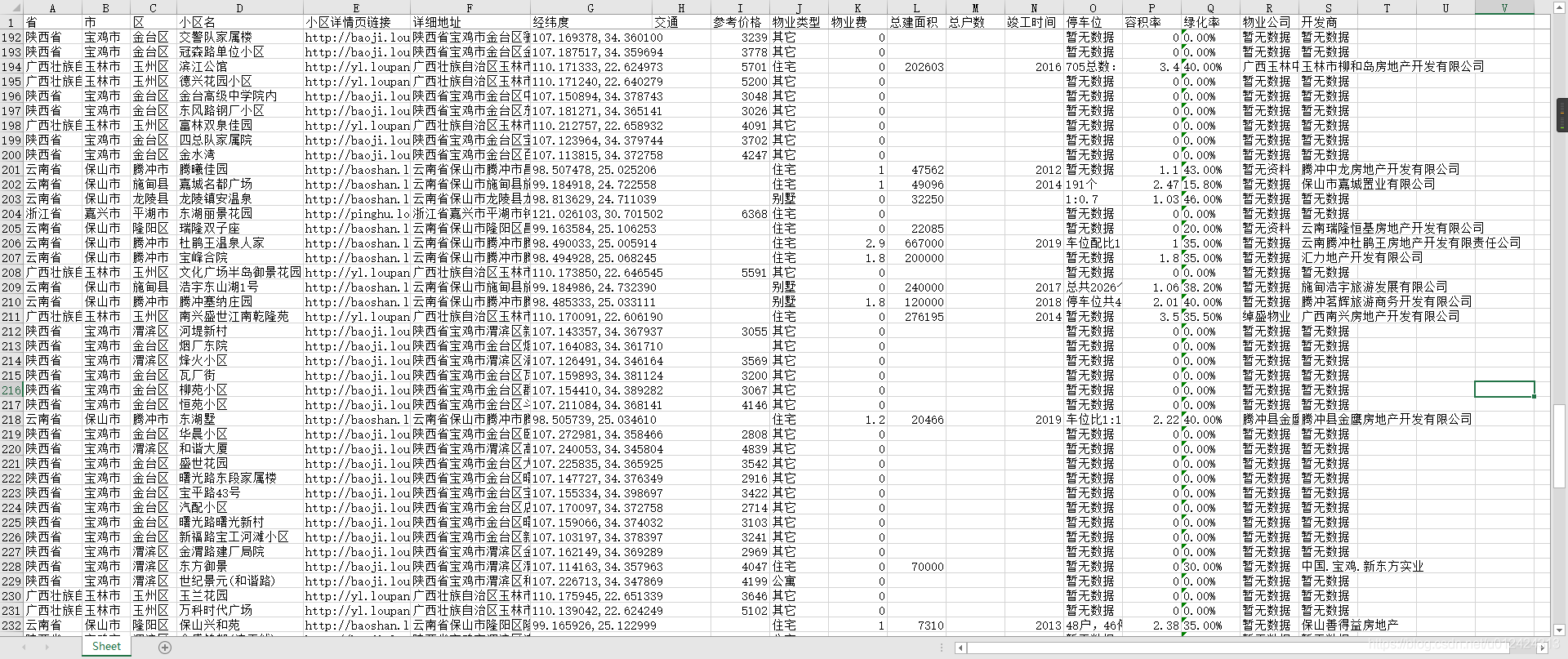
### 配置环境
电脑:win10 64x
python版本:Python 3.7.5 (tags/v3.7.5:5c02a39a0b, Oct 15 2019, 00:11:34) on win32
工具:JetBrains PyCharm Community Edition 2019.2.5 x64
scrapy框架: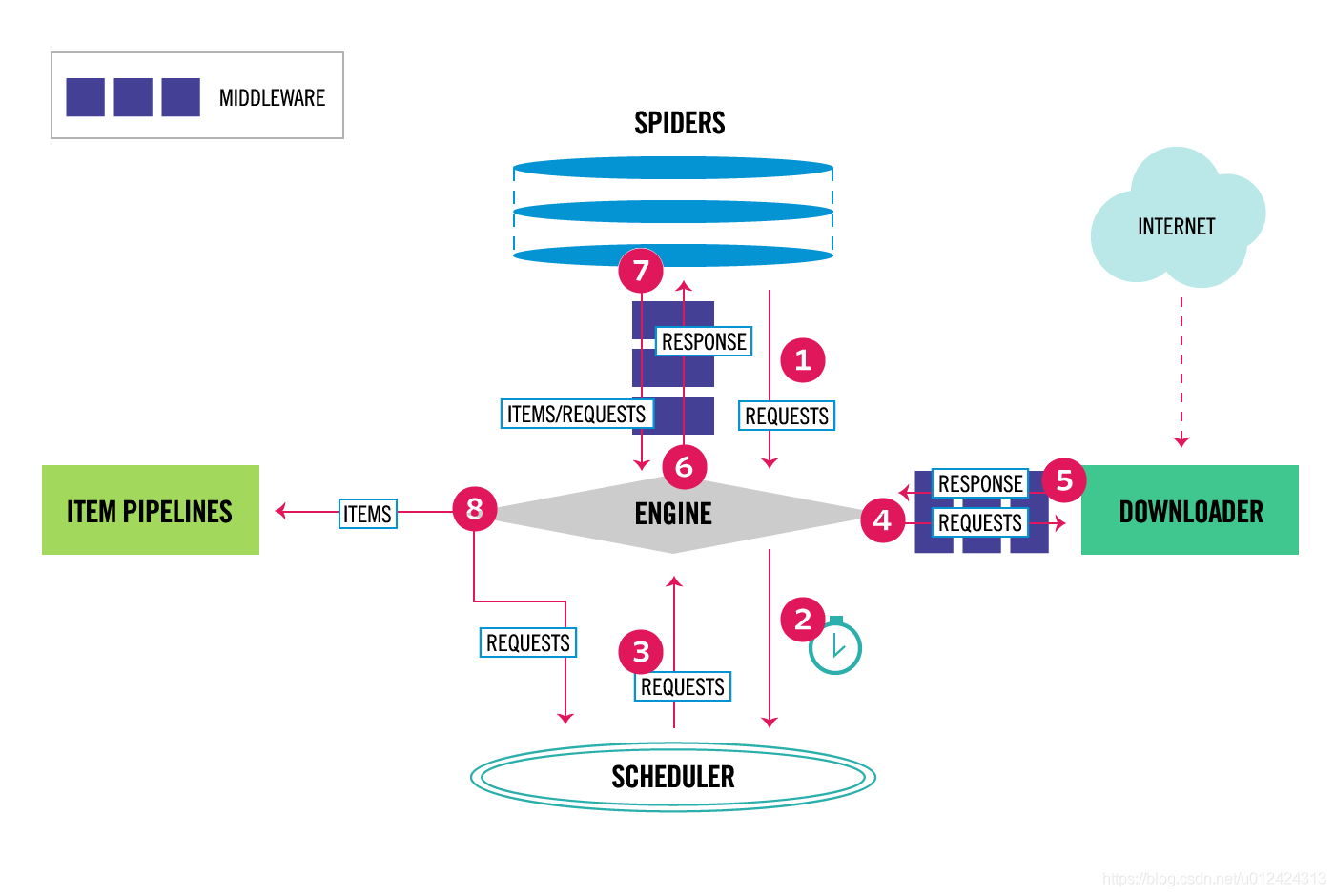
### 分析
为了保证分工明确,提高爬取效率,此次爬取采用python的scrapy框架,因为scrapy自带dupefilters.py去重器,可以不用担心重复爬取。scrapy运行机制如下:
```python
Scrapy运行时,请求发出去的整个流程大概如下:
1.首先爬虫将需要发送请求的url(requests)经引擎交给调度器;
2.排序处理后,经ScrapyEngine,DownloaderMiddlewares(有User_Agent, Proxy代{过}{滤}理)交给Downloader;
3.Downloader向互联网发送请求,并接收下载响应.将响应经ScrapyEngine,可选交给Spiders;
4.Spiders处理response,提取数据并将数据经ScrapyEngine交给ItemPipeline保存;
5.提取url重新经ScrapyEngine交给Scheduler进行下一个循环。直到无Url请求程序停止结束。
```
首先创建scrapy工程
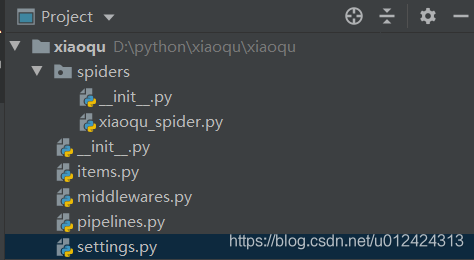
### 爬城市
首先根据网页,获取所有城市的链接
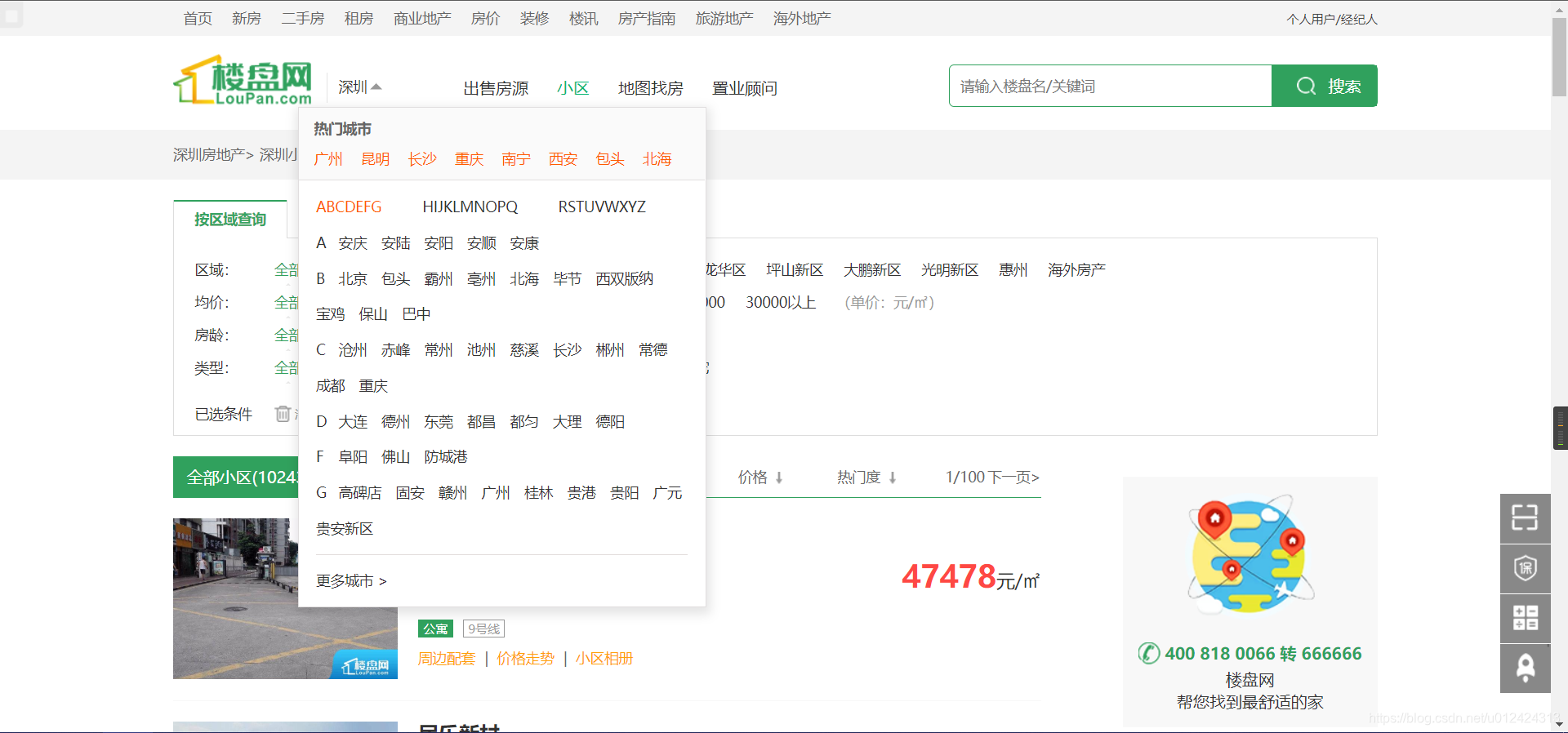
然后就可以多线程的同时爬取所有城市小区,我分析了网页结构,单纯的爬取本页是获取不了城市链接的,城市链接使用的是JavaScript加载的动态数据,既然如此那就获取他的json数据,如下:
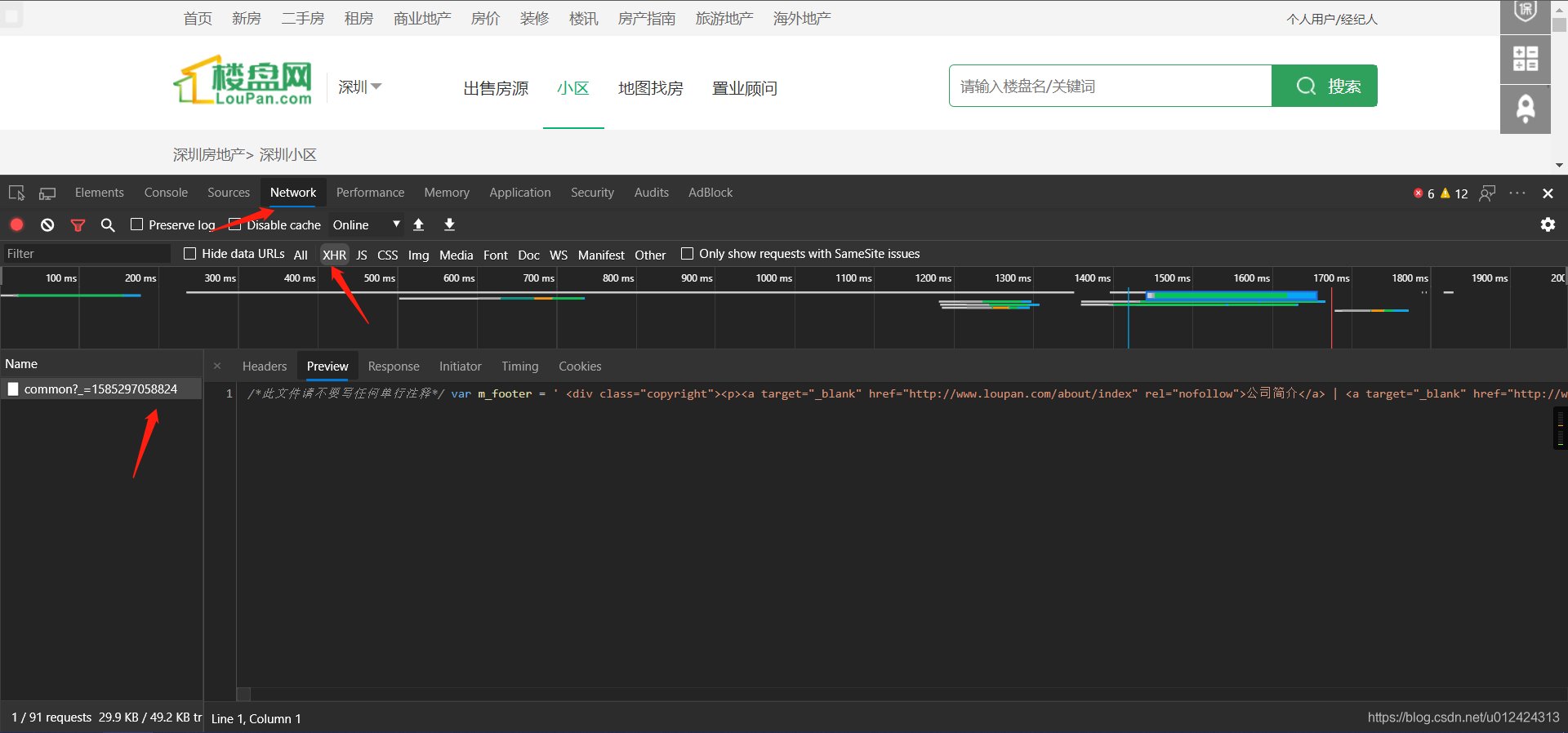
右键获取网址:http://sz.loupan.com/index.php/jsdata/common?_=1579245949843,这是一个json格式列表,我们已经获取所有城市的链接目录,但是这个目录有很多无用网址,所以要进行数据清洗,把无用数据清洗掉。
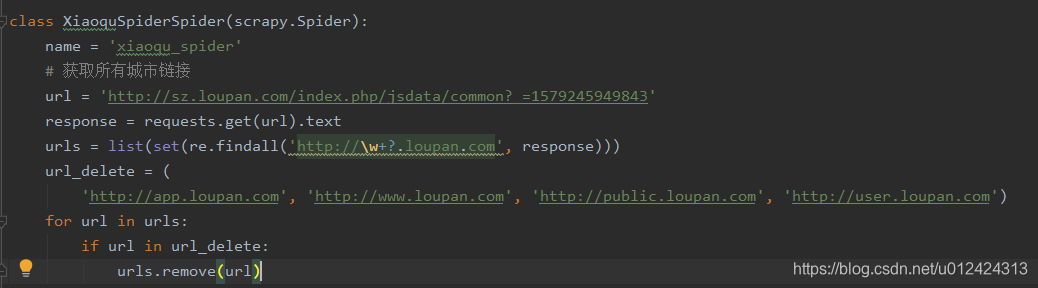
这里使用requests方法获取网页信息,然后使用正则获取链接,再使用for循环清洗数据。
比如深圳市:http://sz.loupan.com/
既然已经获取了所有城市链接,那就开始正式爬取网页上的小区。
### 爬小区
首先在http://sz.loupan.com/community/这一页,我看到,一页有25个小区,然后总共只有100页,
也就是说,如果我按照传统的遍历每一页去爬的话,最多只能爬取2500条数据。
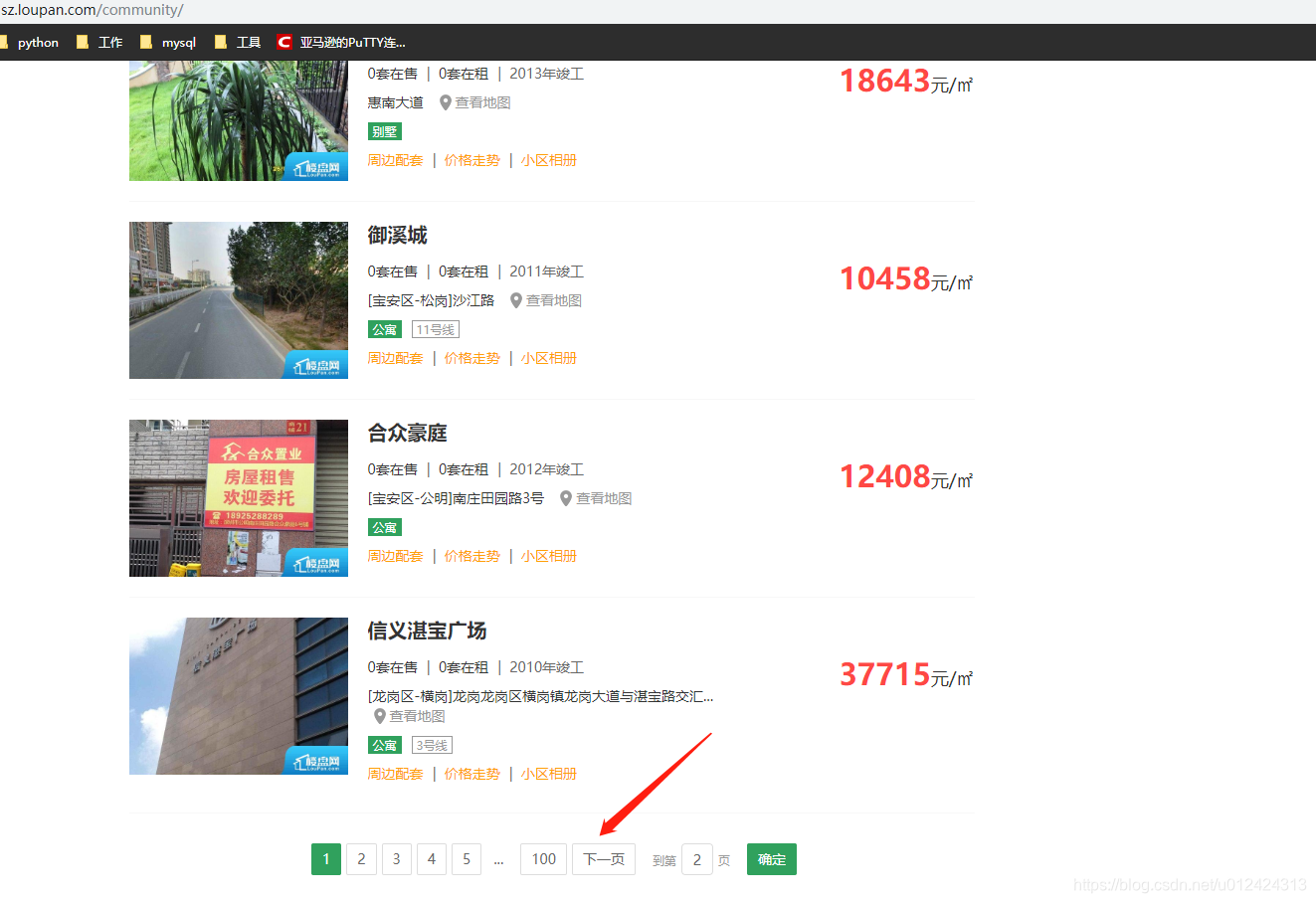
但是,实际上可以看到深圳小区数量是10243
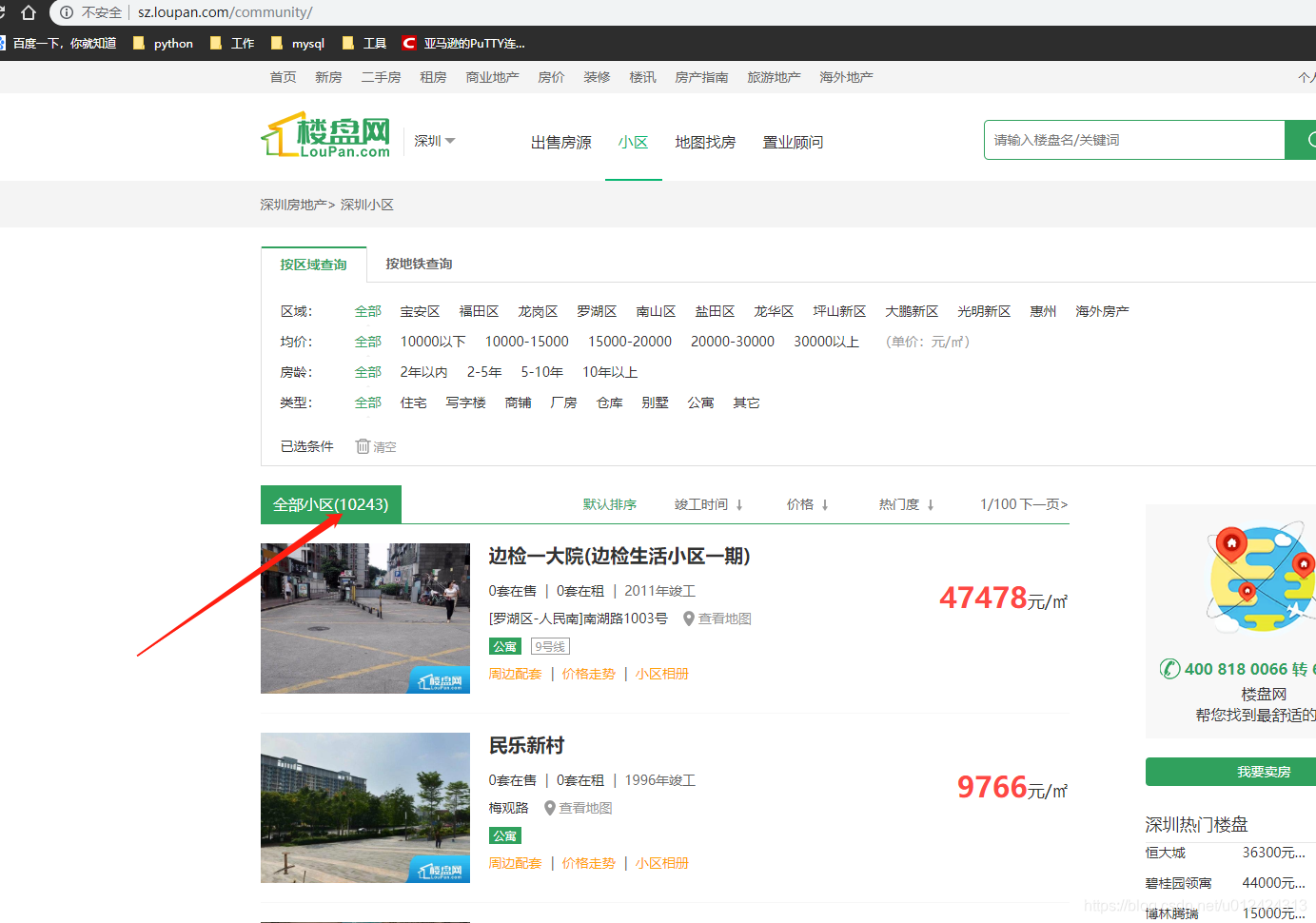
所以,这个时候我转换思路,我随机点开一个小区链接,拉下来可以看到,有一个周边小区推荐
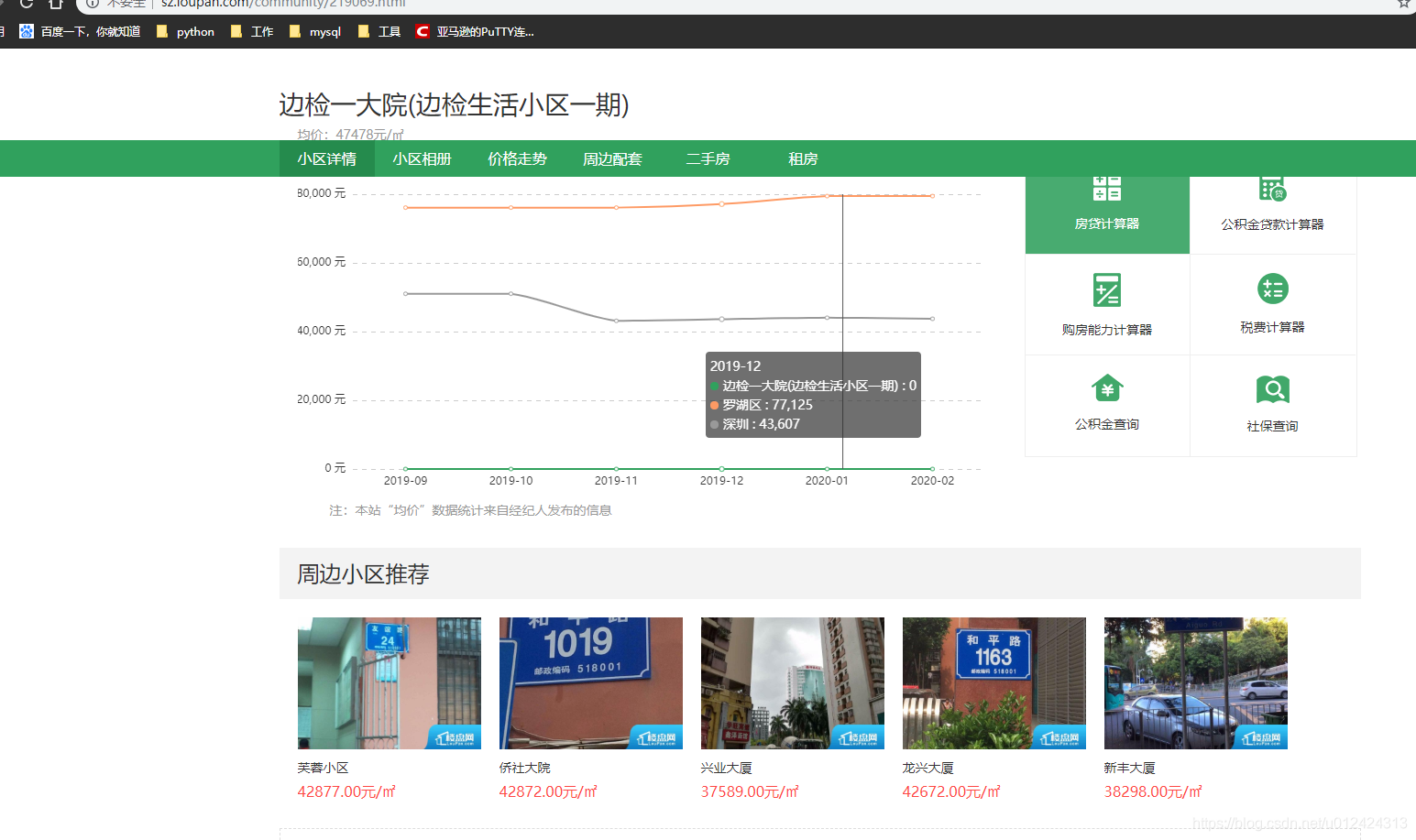
我可以依靠这个,然后不断循环遍历,直至最终爬完所有小区,这也是很多爬虫所运用的方法,包括像爬新浪微博所有用户信息,爬取知乎所有用户信息,都可以从某一个大v出发,然后爬取其关注列表和被关注列表,然后不断循环发散,直至爬完整个新浪用户。
在http://sz.loupan.com/community页面按F12查看深圳小区第一页网页源码,很容易得到网页所有小区链接,25条,然后遍历这25各小区,在每个小区里再遍历周边小区。
好,现在可以爬完所有小区信息了,那问题来了,重复的怎么办,scrapy自带dupefilters.py去重器,可以不用担心重复爬取。
#### scrapy去重原理:
```python
1.Scrapy本身自带有一个中间件;
2.scrapy源码中可以找到一个dupefilters.py去重器;
3.需要将dont_filter设置为False开启去重,默认是True,没有开启去重;
4 .对于每一个url的请求,调度器都会根据请求得相关信息加密得到一个指纹信息,并且将指纹信息和set()集合中的指纹信息进 行 比对,如果set()集合中已经存在这个数据,就不在将这个Request放入队列中;
5.如果set()集合中没有存在这个加密后的数据,就将这个Request对象放入队列中,等待被调度。
```
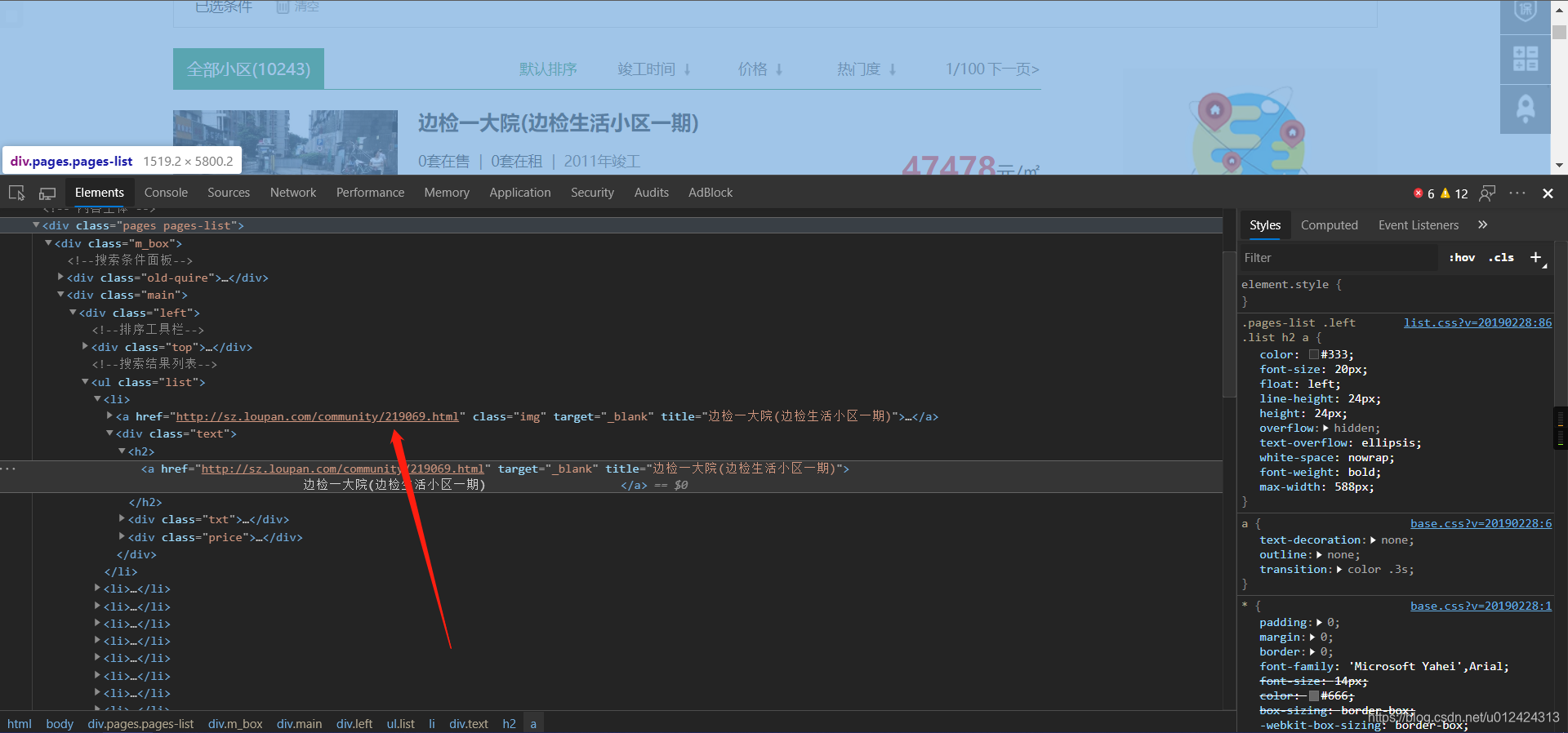
很明显,小区链接就是城市链接+community+小区编号,community是小区的意思。
```python
class XiaoquSpiderSpider(scrapy.Spider):
name = 'xiaoqu_spider'
# 获取所有城市链接
url = 'http://sz.loupan.com/index.php/jsdata/common?_=1579245949843'
response = requests.get(url).text
urls = list(set(re.findall('http://\w+?.loupan.com', response)))
url_delete = (
'http://app.loupan.com', 'http://www.loupan.com', 'http://public.loupan.com', 'http://user.loupan.com')
for url in urls:
if url in url_delete:
urls.remove(url)
```
我这里使用pyquery库的PyQuery方法获取小区链接。
获取链接后,要开始分析小区详情的网页。
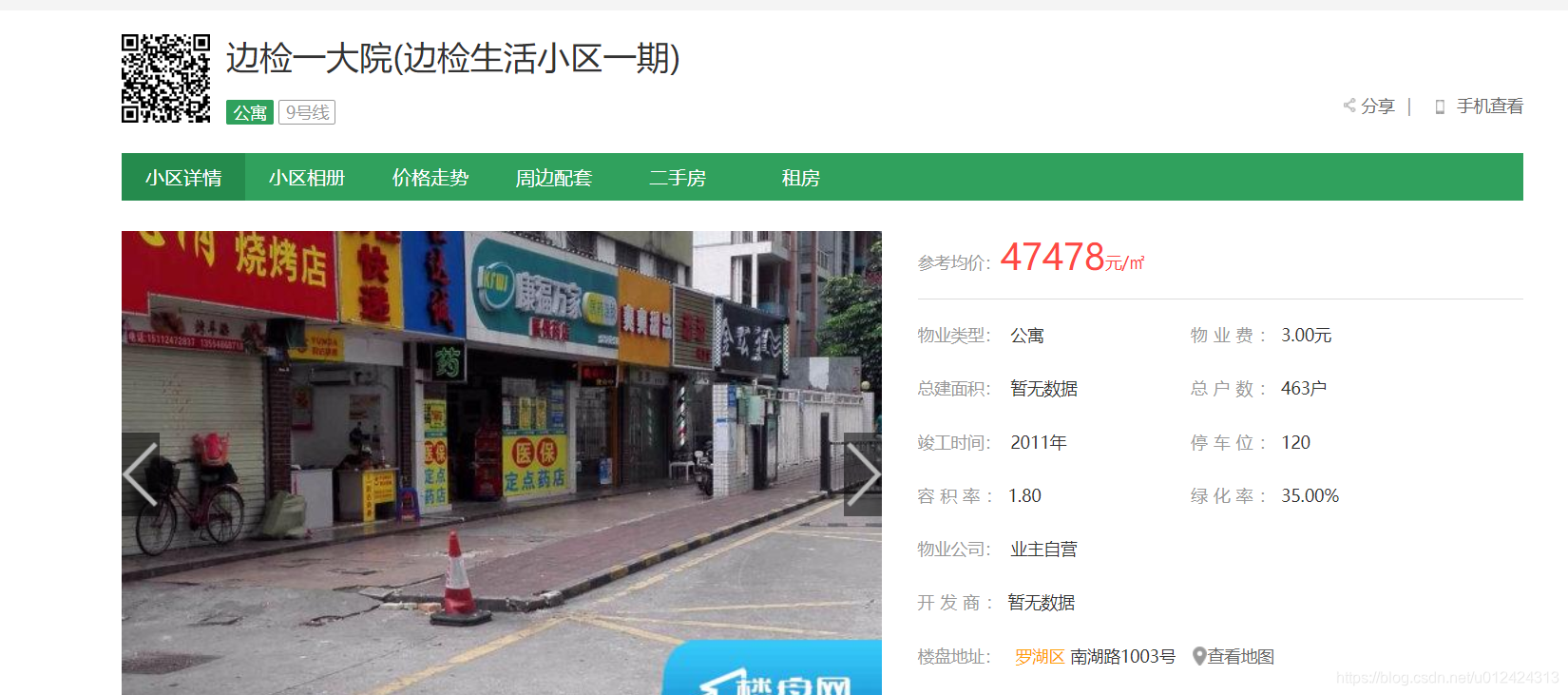
同样方法,F12查看网页源码,然后获取小区的详细信息
```python
def parse(self, response):
doc = pq(response.text)
item = XiaoquItem()
url = doc('.pos > a:nth-child(4)').attr('href')# 小区链接
item['url'] = url# 小区链接
name = doc('.t p').text()# 小区名
item['name'] = name# 小区名
# 根据网页获得小区模糊地址,再通过百度地图API获取经纬度
addres = doc('.text_nr.bug2').text()# 小区地址
citys = doc('.pos > a:nth-child(2)').text()
city = ''.join(re.findall('(\w+)小区', citys)) + '市'
districts = doc('span.font_col_o > a').text()# 所属区
address = city + districts + addres + name# 所属详细地址
# 将地址传入api获取省市区
location = self.location(address)
coord = location['coord']# 经纬度
item['coord'] = coord
province = location['province']# 省
item['province'] = province
city = location['city']# 市
item['city'] = city
district = location['district']# 区
item['district'] = district
item['detail_address'] = province + city + district + addres + name# 详细地址
id = ''.join(re.findall('\d+', url))
around_url = 'http://sz.loupan.com/community/around/' + id + '.html'# 周边信息网址
response = requests.get(around_url)
around_doc = pq(response.text)
traffic = around_doc('.trend > p:nth-child(7)').text()# 交通
item['traffic'] = traffic.replace('m', 'm,')# 交通
prices = doc('div.price > span.dj').text()# 参考价格
if prices == '暂无数据':
price = None
item['price'] = price
else:
price = int(prices)
item['price'] = price
item['property_type'] = doc('ul > li:nth-child(1) > span.text_nr').text()# 物业类型
property_fees = doc('ul > li:nth-child(2) > span.text_nr').text()# 物业费
if property_fees == '暂无数据':
property_fee = None
item['property_fee'] = property_fee
else:
property_fee = float(''.join(re.findall('\d*\.\d*', property_fees)))
item['property_fee'] = property_fee
areas = doc('ul > li:nth-child(3) > span.text_nr').text()# 总建面积
if areas == '暂无数据':
area = None
item['area'] = area
else:
area = int(''.join(re.findall('\d*', areas)))
item['area'] = area
house_counts = doc('ul > li:nth-child(4) > span.text_nr').text()# 总户数
if house_counts == '暂无数据' or house_counts == '':
house_count = None
item['house_count'] = house_count
else:
house_count = int(''.join(re.findall('\d*', house_counts)))
item['house_count'] = house_count
completion_times = doc('ul > li:nth-child(5) > span.text_nr').text()# 竣工时间
if completion_times in ('暂无数据', '', None):
completion_time = None
item['completion_time'] = completion_time
else:
completion_time = int(''.join(re.findall('\d*', completion_times)))
item['completion_time'] = completion_time
item['parking_count'] = doc('ul > li:nth-child(6) > span.text_nr').text()# 停车位
plot_ratios = doc('ul > li:nth-child(7) > span.text_nr').text()# 容积率
if plot_ratios == '暂无数据' or plot_ratios == '':
plot_ratio = None
item['plot_ratio'] = plot_ratio
else:
plot_ratio = float(''.join(re.findall('\d*\.\d*', plot_ratios)))
item['plot_ratio'] = plot_ratio
greening_rates = doc('ul > li:nth-child(8) > span.text_nr').text()# 绿化率
if greening_rates == '暂无数据':
greening_rate = None
item['greening_rate'] = greening_rate
else:
greening_rate = ''.join(re.findall('\d*\.\d*%', greening_rates))
item['greening_rate'] = greening_rate
item['property_company'] = doc('div.ps > p:nth-child(1) > span.text_nr').text()# 物业公司
item['developers'] = doc('div.ps > p:nth-child(2) > span.text_nr').text()# 开发商
yield item
lis = doc('body > div.pages > div.main.esf_xq > div > div.main > div.tj_esf > ul > li')
li_doc = pq(lis).items()
for li in li_doc:
url = li('div.text > a').attr('href')
yield Request(url=url, callback=self.parse)
```
因为要保存到mysql,所以我这里对‘暂无数据’的值进行了数据修改,改成null或者' '。
将所爬取到的数据存储到item模块里面,这是items.py代码:
```python
# -*- coding: utf-8 -*-
# Define here the models for your scraped items
#
# See documentation in:
# https://docs.scrapy.org/en/latest/topics/items.html
import scrapy
class XiaoquItem(scrapy.Item):
collection = table = 'community'
province = scrapy.Field()# 省
city = scrapy.Field()# 市
district = scrapy.Field()# 区
name = scrapy.Field()# 小区名
url = scrapy.Field()# 小区链接
detail_address = scrapy.Field()# 所属详细地址
coord = scrapy.Field()# 经纬度
traffic = scrapy.Field()# 附近交通
price = scrapy.Field()# 参考价格
property_type = scrapy.Field()# 物业类型
property_fee = scrapy.Field()# 物业费
area = scrapy.Field()# 总建面积
house_count = scrapy.Field()# 总户数
completion_time = scrapy.Field()# 竣工时间
parking_count = scrapy.Field()# 停车位
plot_ratio = scrapy.Field()# 容积率
greening_rate = scrapy.Field()# 绿化率
property_company = scrapy.Field()# 物业公司
developers = scrapy.Field()# 开发商
pass
```
这里有一个难点就是获取小区经纬度,因为网页上没有,想要获取经纬度,就得通过调用api来实现,api调用是传入小区详细地址,返回经纬度,我这里使用的是高德地图api,百度太渣了,定位都定到海里去了。。。。
```python
# 调用经高德地图API,获取经纬度与详细地址
def location(self, detail_address):
url = "https://restapi.amap.com/v3/geocode/geo?address=" + detail_address + "&key=你的key码"
response = requests.get(url).json()
geocodes = response['geocodes']
for geocode in geocodes:
coord = geocode['location']
province = geocode['province']
city = geocode['city']
district = geocode['district']
local = {'coord': coord, 'province': province, 'city': city, 'district': district}
return local
```
可以去高德地图申请一个开发者账号,然后认证一下,每天有30万次配额,完全够用
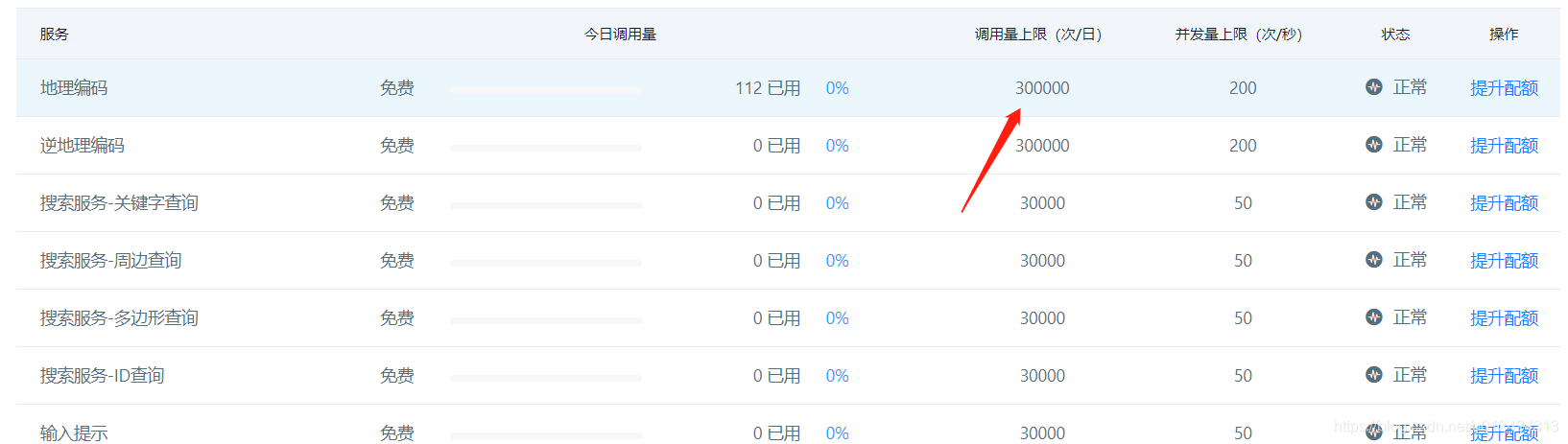
基本上小区的所有信息就分析完毕。
### 保存
python scrapy框架的保存是很方便的,使用的是项目管道(ITEM PIPLINES)来保存,可以在这里进行数据清洗和数据存储,我使用的是excel和mysql来保存,pipelines.py代码:
```python
# -*- coding: utf-8 -*-
# Define your item pipelines here
#
# Don't forget to add your pipeline to the ITEM_PIPELINES setting
# See: https://docs.scrapy.org/en/latest/topics/item-pipeline.html
from openpyxl import Workbook
import pymysql
class XiaoquPipeline(object):
def process_item(self, item, spider):
return item
class ExcelPipeline(object):
def __init__(self):
self.wb = Workbook()
self.ws = self.wb.active
self.ws.append(['省', '市', '区', '小区名', '小区详情页链接', '详细地址', '经纬度', '交通',
'参考价格', '物业类型', '物业费', '总建面积', '总户数', '竣工时间', '停车位', '容积率', '绿化率',
'物业公司', '开发商'])
def process_item(self, item, spider):
line = , item['city'], item['district'], item['name'], item['url'], item['detail_address'],
item['coord'], item['traffic'], item['price'], item['property_type'],
item['property_fee'], item['area'], item['house_count'], item['completion_time'], item['parking_count'],
item['plot_ratio'], item['greening_rate'], item['property_company'], item['developers']]
self.ws.append(line)
# keys = spider.settings.get('KEYS')
self.wb.save('../小区' + '.xlsx')
return item
class MysqlPipeline():
def __init__(self, host, database, user, password, port):
self.host = host
self.database = database
self.user = user
self.password = password
self.port = port
@classmethod
def from_crawler(cls, crawler):
return cls(
host=crawler.settings.get('MYSQL_HOST'),
database=crawler.settings.get('MYSQL_DATABASE'),
user=crawler.settings.get('MYSQL_USER'),
password=crawler.settings.get('MYSQL_PASSWORD'),
port=crawler.settings.get('MYSQL_PORT'),
)
def open_spider(self, spider):
self.db = pymysql.connect(self.host, self.user, self.password, self.database, charset='utf8',
port=self.port)
self.cursor = self.db.cursor()
def close_spider(self, spider):
self.db.close()
def process_item(self, item, spider):
# print(item['raw_title'])
data = dict(item)
keys = ', '.join(data.keys())
values = ', '.join(['%s'] * len(data))
sql = 'insert into %s (%s) values (%s)' % (item.table, keys, values)
self.cursor.execute(sql, tuple(data.values()))
self.db.commit()
return item
```
这里调用了openpyxl来保存至excel和pymysql保存至mysql,这里excel是自动在上一级目录创建一个excel文档,并保存数据。
mysql的话需要服务器,账号密码等配置信息,这些信息在settings里可以设置。
我这里是保存到一个community的数据库的community表里面,所以大家运行的时候需要注意,或者修改成自己的数据库名就好。
保存至mysql之前需要在mysql创建相应表,创建代码:
```sql
CREATE TABLE `community`.`community`(
`id` int(11) UNSIGNED NOT NULL AUTO_INCREMENT,
`province` varchar(255) CHARACTER SET utf8mb4 COLLATE utf8mb4_general_ci NULL DEFAULT NULL COMMENT '省',
`city` varchar(255) CHARACTER SET utf8mb4 COLLATE utf8mb4_general_ci NULL DEFAULT NULL COMMENT '所属市',
`district` varchar(255) CHARACTER SET utf8mb4 COLLATE utf8mb4_general_ci NULL DEFAULT NULL COMMENT '所属区',
`name` varchar(255) CHARACTER SET utf8mb4 COLLATE utf8mb4_general_ci NULL DEFAULT NULL COMMENT '小区名',
`url` varchar(255) CHARACTER SET utf8mb4 COLLATE utf8mb4_general_ci NULL DEFAULT NULL COMMENT '小区链接',
`detail_address` varchar(255) CHARACTER SET utf8mb4 COLLATE utf8mb4_general_ci NULL DEFAULT NULL COMMENT '详细地址',
`coord` varchar(255) CHARACTER SET utf8mb4 COLLATE utf8mb4_general_ci NULL DEFAULT NULL COMMENT '经纬度',
`traffic` varchar(555) CHARACTER SET utf8mb4 COLLATE utf8mb4_general_ci NULL DEFAULT NULL COMMENT '交通',
`price` int(10) NULL DEFAULT NULL COMMENT '价格',
`property_type` varchar(255) CHARACTER SET utf8mb4 COLLATE utf8mb4_general_ci NULL DEFAULT NULL COMMENT '物业类型',
`property_fee` decimal(10, 2) NULL DEFAULT NULL COMMENT '物业价格',
`area` int(20) NULL DEFAULT NULL COMMENT '面积',
`house_count` int(10) NULL DEFAULT NULL COMMENT '户数',
`completion_time` int(4) NULL DEFAULT NULL COMMENT '竣工时间',
`parking_count` varchar(255) CHARACTER SET utf8mb4 COLLATE utf8mb4_general_ci NULL DEFAULT NULL COMMENT '车位数',
`plot_ratio` decimal(10, 2) NULL DEFAULT NULL COMMENT '容积率',
`greening_rate` varchar(10) CHARACTER SET utf8mb4 COLLATE utf8mb4_general_ci NULL DEFAULT NULL COMMENT '绿化率',
`property_company` varchar(255) CHARACTER SET utf8mb4 COLLATE utf8mb4_general_ci NULL DEFAULT NULL COMMENT '物业公司',
`developers` varchar(255) CHARACTER SET utf8mb4 COLLATE utf8mb4_general_ci NULL DEFAULT NULL COMMENT '开发商',
`create_time` datetime(0) NULL DEFAULT CURRENT_TIMESTAMP(0) ON UPDATE CURRENT_TIMESTAMP(0) COMMENT '插入时间',
PRIMARY KEY (`id`) USING BTREE
) ENGINE = InnoDB AUTO_INCREMENT = 3080 CHARACTER SET = utf8mb4 COLLATE = utf8mb4_general_ci ROW_FORMAT = Dynamic;
```
### 设置
现在万事俱备,还差一个settings.py,配置信息,如何配置的问题。
首先ROBOTSTXT_OBEY,这是个啥呢,
```python
爬虫协议,即 robots 协议,也叫机器人协议
它用来限定爬虫程序可以爬取的内容范围
通常写在 robots.txt 文件中
该文件保存在网站的服务器上
爬虫程序访问网站时首先查看此文件
在 scrapy 项目的 settings.py 文件中
默认 ROBOTSTXT_OBEY = True ,即遵守此协议
当爬取内容不符合该协议且仍要爬取时
设置 ROBOTSTXT_OBEY = False ,不遵守此协议
```
我们是爬虫,所以肯定要FALSE的,不然爬不起来。
然后是下载中间器DOWNLOADER_MIDDLEWARES,这个是默认是屏蔽的,需要我们解除屏蔽,不然没法把数据下载下来。
接下来就是ITEM_PIPELINES,项目管道的配置,需要和管道的下载器名对应,不然不知道你保存到哪儿。
```python
ITEM_PIPELINES = {
# 'xiaoqu.pipelines.XiaoquPipeline': 300,
'xiaoqu.pipelines.ExcelPipeline': 301,
'xiaoqu.pipelines.MysqlPipeline': 302,
}
```
默认是只有第一行,我们添加excel和mysql,如果没有安装mysql的小伙伴,可以屏蔽掉mysql管道。
我的spider里面调用了一个请求头headers,因为一般的网站都有反爬措施,这个网站暂时没有,但是也加上。还有之前说过的mysql配置信息
```python
headers = {
'Accept': 'text/html,application/xhtml+xml,application/xml;q=0.9,image/webp,image/apng,*/*;q=0.8,application/signed-exchange;v=b3',
'Accept-Encoding': 'gzip, deflate',
'Accept-Language': 'zh-CN,zh;q=0.9,en;q=0.8',
'Cache-Control': 'max-age=0',
'Connection': 'keep-alive',
'Cookie': '__customer_trace_id=B8D70080-5CA9-45D9-995E-F3DAB0EC0D1E; PHPSESSID=4qipinajmn8q9pvmkdcfpp3fh1; Hm_lvt_c07a5cf91cdac070faa1e701f45995a8=1577343206,1577347508; AGL_USER_ID=e79f0249-d96f-4a98-837a-398d4bb287b8; Hm_lvt_15e5e51b14c8efd1f1488ea51faa1172=1577347593,1577347761,1577771483,1578894243; loadDomain=http%3A%2F%2Fsz.loupan.com%2F; Hm_lvt_2c0f8f2133c1fc1d09538a565dd8d6c8=1577343206,1577347508,1579223144; Hm_lpvt_15e5e51b14c8efd1f1488ea51faa1172=1579240756; loupan_user_session=a%3A5%3A%7Bs%3A10%3A%22session_id%22%3Bs%3A32%3A%22622161d9507e199454d3eb844eb97b5d%22%3Bs%3A10%3A%22ip_address%22%3Bs%3A13%3A%22121.15.170.60%22%3Bs%3A10%3A%22user_agent%22%3Bs%3A115%3A%22Mozilla%2F5.0+%28Windows+NT+10.0%3B+Win64%3B+x64%29+AppleWebKit%2F537.36+%28KHTML%2C+like+Gecko%29+Chrome%2F78.0.3904.108+Safari%2F537.36%22%3Bs%3A13%3A%22last_activity%22%3Bi%3A1579241140%3Bs%3A9%3A%22user_data%22%3Bs%3A0%3A%22%22%3B%7Dd2512f1f510eeb07c62f3db5e3ee5796; Hm_lpvt_2c0f8f2133c1fc1d09538a565dd8d6c8=1579241158; Hm_lpvt_c07a5cf91cdac070faa1e701f45995a8=1579241159',
'Host': 'sz.loupan.com',
'Upgrade-Insecure-Requests': 1,
'User-Agent': 'Mozilla/5.0 (Windows NT 10.0; Win64; x64) AppleWebKit/537.36 (KHTML, like Gecko) Chrome/78.0.3904.108 Safari/537.36'
}
#LOG_LEVEL = 'INFO'
RETRY_ENABLED = False
#DOWNLOAD_TIMEOUT = 10
MYSQL_HOST = 'localhost'
MYSQL_DATABASE = 'community'
MYSQL_USER = 'root'
MYSQL_PASSWORD = '123456'
MYSQL_PORT = 3306
```
这样配置的话,基本上整个项目就完成了,但是要提升效率,提高爬取速度,再配置以下信息
```python
# Configure maximum concurrent requests performed by Scrapy (default: 16)
CONCURRENT_REQUESTS = 100
DOWNLOAD_DELAY = 0
# The download delay setting will honor only one of:
CONCURRENT_REQUESTS_PER_DOMAIN = 100
CONCURRENT_REQUESTS_PER_IP = 100
# Disable cookies (enabled by default)
COOKIES_ENABLED = False
RETRY_ENABLED = False
```
使用多线程,低延时,拒绝重试等等,反正不反爬,都无所谓啦,settings.py完整代码:
```python
# -*- coding: utf-8 -*-
# Scrapy settings for xiaoqu project
#
# For simplicity, this file contains only settings considered important or
# commonly used. You can find more settings consulting the documentation:
#
# https://docs.scrapy.org/en/latest/topics/settings.html
# https://docs.scrapy.org/en/latest/topics/downloader-middleware.html
# https://docs.scrapy.org/en/latest/topics/spider-middleware.html
BOT_NAME = 'xiaoqu'
SPIDER_MODULES = ['xiaoqu.spiders']
NEWSPIDER_MODULE = 'xiaoqu.spiders'
# Crawl responsibly by identifying yourself (and your website) on the user-agent
USER_AGENT = 'Mozilla/5.0 (Windows NT 6.1; WOW64) AppleWebKit/537.36 (KHTML, like Gecko) Chrome/65.0.3325.162 Safari/537.36'
# Obey robots.txt rules
ROBOTSTXT_OBEY = False
# Configure maximum concurrent requests performed by Scrapy (default: 16)
CONCURRENT_REQUESTS = 100
# Configure a delay for requests for the same website (default: 0)
# See https://docs.scrapy.org/en/latest/topics/settings.html#download-delay
# See also autothrottle settings and docs
DOWNLOAD_DELAY = 0
# The download delay setting will honor only one of:
CONCURRENT_REQUESTS_PER_DOMAIN = 100
CONCURRENT_REQUESTS_PER_IP = 100
# Disable cookies (enabled by default)
COOKIES_ENABLED = False
# Disable Telnet Console (enabled by default)
# TELNETCONSOLE_ENABLED = False
# Override the default request headers:
# DEFAULT_REQUEST_HEADERS = {
# 'Accept': 'text/html,application/xhtml+xml,application/xml;q=0.9,*/*;q=0.8',
# 'Accept-Language': 'en',
# }
# Enable or disable spider middlewares
# See https://docs.scrapy.org/en/latest/topics/spider-middleware.html
# SPIDER_MIDDLEWARES = {
# 'xiaoqu.middlewares.XiaoquSpiderMiddleware': 543,
# }
# Enable or disable downloader middlewares
# See https://docs.scrapy.org/en/latest/topics/downloader-middleware.html
DOWNLOADER_MIDDLEWARES = {
'xiaoqu.middlewares.XiaoquDownloaderMiddleware': 543,
}
# Enable or disable extensions
# See https://docs.scrapy.org/en/latest/topics/extensions.html
# EXTENSIONS = {
# 'scrapy.extensions.telnet.TelnetConsole': None,
# }
# Configure item pipelines
# See https://docs.scrapy.org/en/latest/topics/item-pipeline.html
ITEM_PIPELINES = {
# 'xiaoqu.pipelines.XiaoquPipeline': 300,
'xiaoqu.pipelines.ExcelPipeline': 301,
'xiaoqu.pipelines.MysqlPipeline': 302,
}
# Enable and configure the AutoThrottle extension (disabled by default)
# See https://docs.scrapy.org/en/latest/topics/autothrottle.html
# AUTOTHROTTLE_ENABLED = True
# The initial download delay
# AUTOTHROTTLE_START_DELAY = 5
# The maximum download delay to be set in case of high latencies
# AUTOTHROTTLE_MAX_DELAY = 60
# The average number of requests Scrapy should be sending in parallel to
# each remote server
# AUTOTHROTTLE_TARGET_CONCURRENCY = 1.0
# Enable showing throttling stats for every response received:
# AUTOTHROTTLE_DEBUG = False
# Enable and configure HTTP caching (disabled by default)
# See https://docs.scrapy.org/en/latest/topics/downloader-middleware.html#httpcache-middleware-settings
# HTTPCACHE_ENABLED = True
# HTTPCACHE_EXPIRATION_SECS = 0
# HTTPCACHE_DIR = 'httpcache'
# HTTPCACHE_IGNORE_HTTP_CODES = []
# HTTPCACHE_STORAGE = 'scrapy.extensions.httpcache.FilesystemCacheStorage'
headers = {
'Accept': 'text/html,application/xhtml+xml,application/xml;q=0.9,image/webp,image/apng,*/*;q=0.8,application/signed-exchange;v=b3',
'Accept-Encoding': 'gzip, deflate',
'Accept-Language': 'zh-CN,zh;q=0.9,en;q=0.8',
'Cache-Control': 'max-age=0',
'Connection': 'keep-alive',
'Cookie': '__customer_trace_id=B8D70080-5CA9-45D9-995E-F3DAB0EC0D1E; PHPSESSID=4qipinajmn8q9pvmkdcfpp3fh1; Hm_lvt_c07a5cf91cdac070faa1e701f45995a8=1577343206,1577347508; AGL_USER_ID=e79f0249-d96f-4a98-837a-398d4bb287b8; Hm_lvt_15e5e51b14c8efd1f1488ea51faa1172=1577347593,1577347761,1577771483,1578894243; loadDomain=http%3A%2F%2Fsz.loupan.com%2F; Hm_lvt_2c0f8f2133c1fc1d09538a565dd8d6c8=1577343206,1577347508,1579223144; Hm_lpvt_15e5e51b14c8efd1f1488ea51faa1172=1579240756; loupan_user_session=a%3A5%3A%7Bs%3A10%3A%22session_id%22%3Bs%3A32%3A%22622161d9507e199454d3eb844eb97b5d%22%3Bs%3A10%3A%22ip_address%22%3Bs%3A13%3A%22121.15.170.60%22%3Bs%3A10%3A%22user_agent%22%3Bs%3A115%3A%22Mozilla%2F5.0+%28Windows+NT+10.0%3B+Win64%3B+x64%29+AppleWebKit%2F537.36+%28KHTML%2C+like+Gecko%29+Chrome%2F78.0.3904.108+Safari%2F537.36%22%3Bs%3A13%3A%22last_activity%22%3Bi%3A1579241140%3Bs%3A9%3A%22user_data%22%3Bs%3A0%3A%22%22%3B%7Dd2512f1f510eeb07c62f3db5e3ee5796; Hm_lpvt_2c0f8f2133c1fc1d09538a565dd8d6c8=1579241158; Hm_lpvt_c07a5cf91cdac070faa1e701f45995a8=1579241159',
'Host': 'sz.loupan.com',
'Upgrade-Insecure-Requests': 1,
'User-Agent': 'Mozilla/5.0 (Windows NT 10.0; Win64; x64) AppleWebKit/537.36 (KHTML, like Gecko) Chrome/78.0.3904.108 Safari/537.36'
}
#LOG_LEVEL = 'INFO'
RETRY_ENABLED = False
#DOWNLOAD_TIMEOUT = 10
MYSQL_HOST = 'localhost'
MYSQL_DATABASE = 'community'
MYSQL_USER = 'root'
MYSQL_PASSWORD = '123456'
MYSQL_PORT = 3306
```
### 运行
在控制台进入xiaoqu/spiders目录,然后使用scrapy crawl xiaoqu_spider运行,这个xiaoqu_spider.py是主要的爬取代码
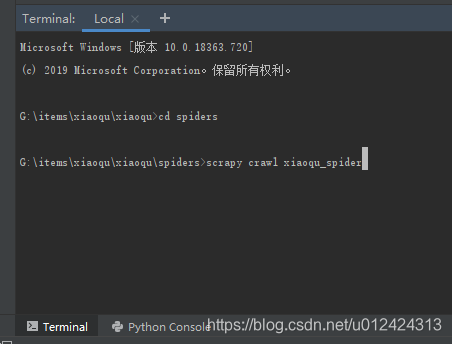
回车运行:
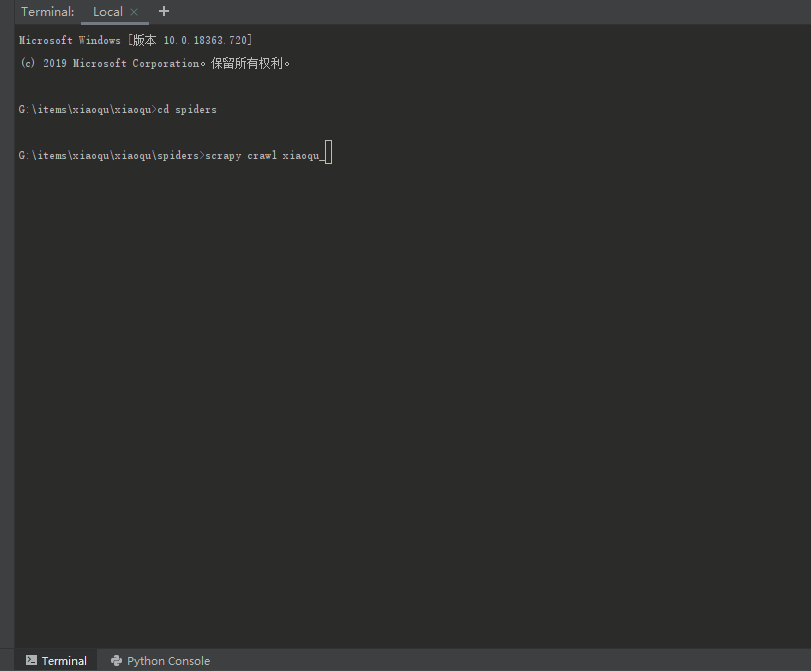
到这里基本上就完成了一个爬取任务,其中有许多字符类型之类的错误,我就没有去细究了,有时候会出现某个字段报错。
打包后的exe文件,因为可能有些小伙伴需要修改配置文件,所以没有打包成一个单exe文件,双击蜘蛛侠图标运行
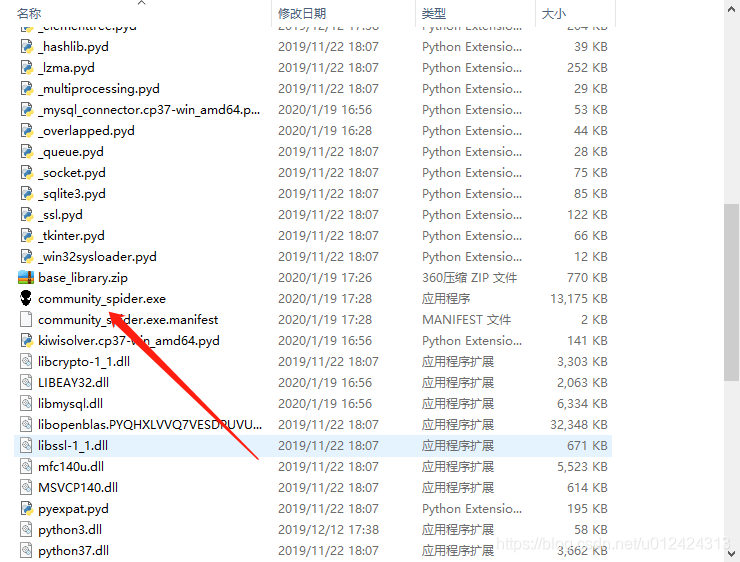
运行图示:
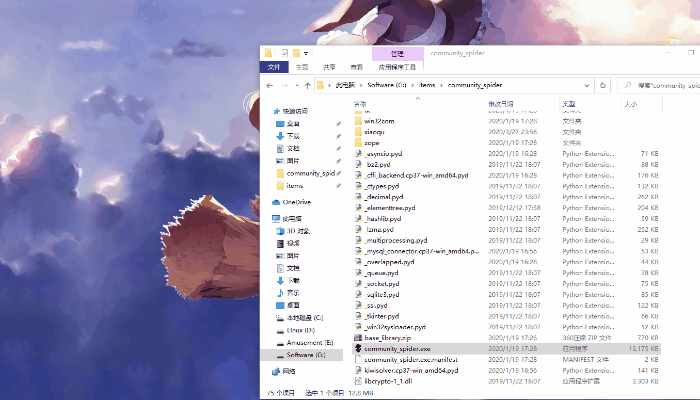
配置文件修改路径:community_spider\xiaoqu\settings
源码github链接:https://github.com/yishaolingxian/community_spiders
打包的exe链接:https://www.lanzouj.com/iapt67c 真的很详细很厉害,我昨天刚开始0基础学python,目标就是学点技术以后会用爬虫,真的太牛掰了,那么多信息,自动爬下来 写的非常详细,感谢。一直还没有用过scrapy这种程序。 看着有点晕,下存一下 写得很好很详细!我先学习,后面再来看成都的效果 为什么要扒宝鸡市的 一直只会用requests的路过 目前只会request ,一直想学scrapy,多谢! 写得非常好, 学习了, 谢谢分享
页:
[1]
2